Ensemble‐based Reconstructed Forcing (ERF) for regional climate modeling: Attaining the performance at a fraction of cost
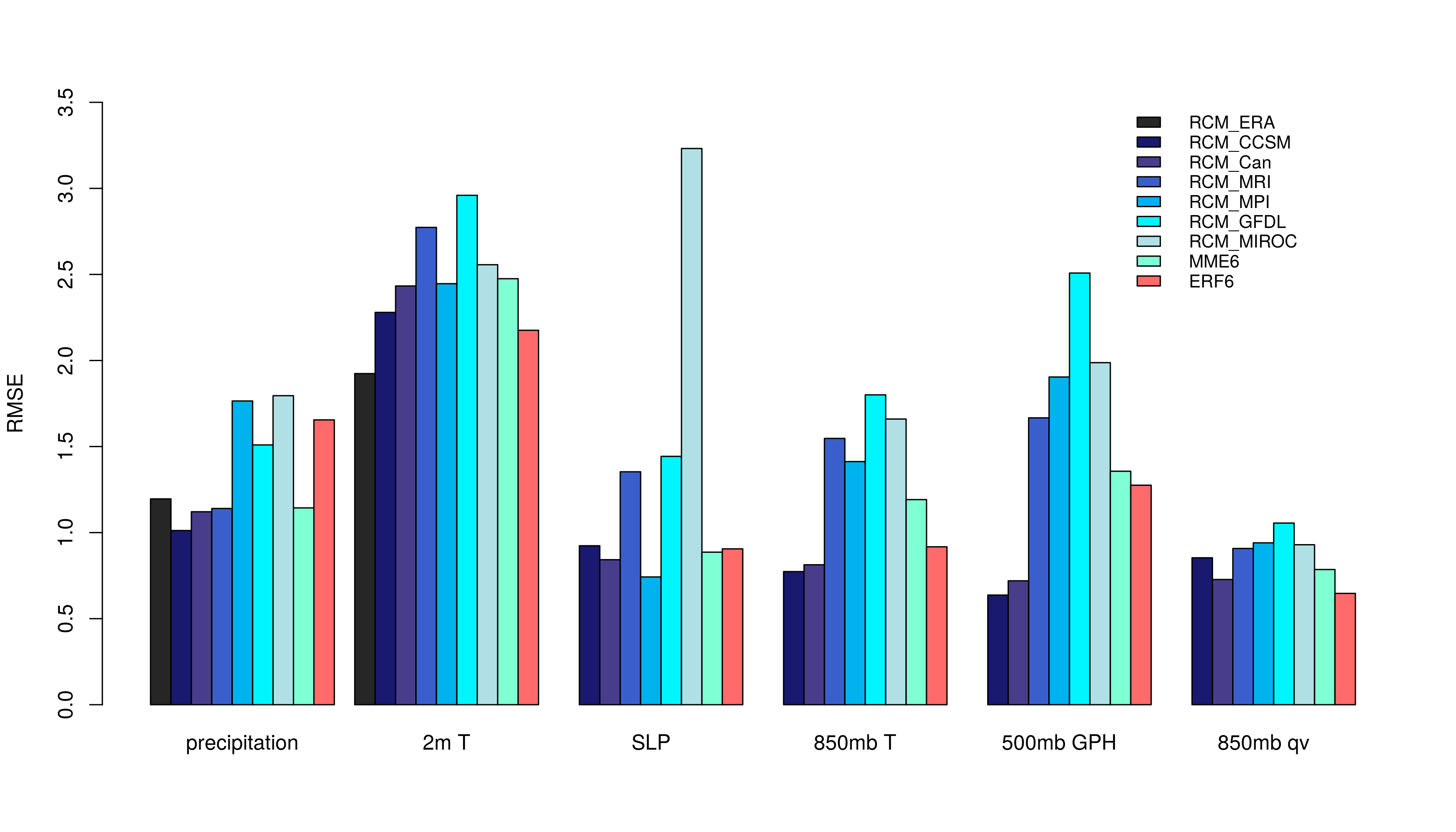
Multimodel ensemble (MME) is the most widely used method to address various sources of uncertainty in climate simulations. However, the conventional MME approach can create physical inconsistencies between climate variables. It is also computationally inhibiting when using regional climate models (RCMs) due to uncertainties originating from both RCMs and the driving global climate models (GCMs) which must be accounted for. Here we proposed the Ensemble‐based Reconstructed Forcing (ERF) approach which derives the RCM's initial and boundary conditions from the ensemble average of multiple GCMs. Using ensembles of up to six GCMs as examples, we show that the ERF approach with a single RCM integration performs comparably to the MME average in bias reduction. The ERF simulation provides unaltered solutions to the RCM's underlying equations, making it theoretically superior to the conventional MME approach which averages multiple solutions and may result in a physically implausible climate state.
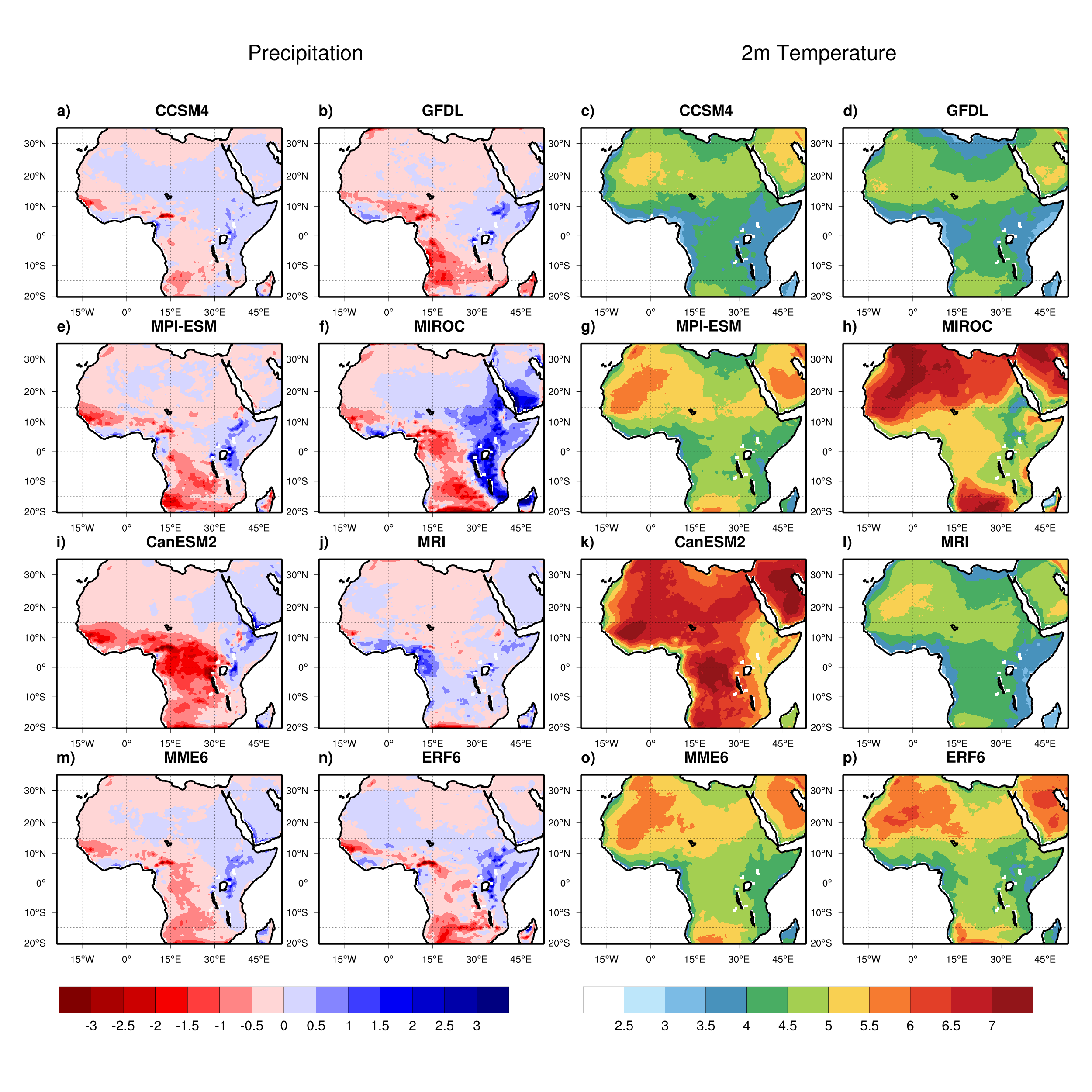
Here are some of the tools and techniques I used in this project:
- Ensemble modeling, Ensemble analysis, exploratory data analysis, geospatial data analysis
- Model verification, model skill, bias-variability tradeoff, uncertainty
- Climate projections, CMIP5 simulations, Regional Climate Models, Lateral Boundary Conditions
You can find more information about this project at:
Erfanian, Amir, Guiling Wang, Lori Fomenko, and Miao Yu. "Ensemble‐based Reconstructed Forcing (ERF) for regional climate modeling: Attaining the performance at a fraction of cost." Geophysical Research Letters 44, no. 7 (2017): 3290-3298.